Deborah
I am Deborah, a computer vision engineer and AI innovator specializing in transforming raw video data into actionable insights through intelligent content analysis. Over the past decade, I have developed AI systems that automatically generate precise tags, summaries, and contextual narratives for videos across industries—from media and entertainment to security and education. My work bridges cutting-edge machine learning with real-world applications, empowering organizations to unlock the hidden value of video content at scale. Below is a detailed overview of my expertise, transformative projects, and vision for the future of AI-driven video intelligence.
1. Academic and Professional Foundations
Education:
Ph.D. in Computer Vision and Natural Language Processing (2024), Stanford University, Dissertation: "Multimodal Fusion Models for Real-Time Video Summarization and Semantic Tagging."
M.Sc. in Artificial Intelligence (2022), Carnegie Mellon University, focused on deep learning architectures for large-scale video analysis.
B.S. in Computer Science (2020), MIT, with a thesis on self-supervised learning for video representation.
Career Milestones:
Lead AI Architect at VisionAI Labs (2023–Present): Developed VidGenius, an end-to-end platform automating video tagging and summarization for 500+ enterprises, reducing manual annotation costs by 70%.
Senior Researcher at Meta AI (2021–2023): Created ClipSense, a transformer-based model generating context-aware video summaries with 93% accuracy, deployed across Instagram Reels and Facebook Watch.
2. Technical Expertise and Innovations
Core Competencies
Video Content Recognition:
Designed SceneNet, a 3D CNN architecture detecting objects, actions, and scenes in videos with 97% precision, even in low-light or cluttered environments.
Engineered TemporalAttn, a hybrid model combining attention mechanisms and RNNs to track temporal dependencies in long-form videos (e.g., lectures, sports events).
Automated Tagging and Metadata Generation:
Built TagMaster, a multimodal system integrating visual, audio, and textual cues to generate SEO-friendly tags, boosting content discoverability by 50%.
Developed EmoTag, an AI analyzing facial expressions and speech tones to assign emotional labels (e.g., "joy," "suspense") for personalized content recommendations.
Summarization and Narrative Generation:
Created SummAIze, a BERT-based model producing concise video summaries in 10+ languages, tailored for educational and corporate training content.
Pioneered StoryFlow, an AI reconstructing fragmented video clips into coherent narratives for documentary filmmakers and news agencies.
Ethical and Inclusive AI
Bias Mitigation:
Launched FairFrame, a toolkit auditing video datasets for demographic biases (e.g., underrepresentation of certain ethnicities) and retraining models for equitable tagging.
Privacy Preservation:
Designed PrivacyGuard, an on-device AI anonymizing faces and voices in real-time video analysis to comply with GDPR and CCPA regulations.
3. High-Impact Deployments
Project 1: "Smart Newsroom 2024" (Reuters)
Deployed SummAIze and TagMaster for Reuters’ global video journalism:
Innovations:
Real-Time Event Tagging: Automated tagging of breaking news videos (e.g., protests, disasters) within 15 seconds of upload.
Multilingual Summaries: Generated 1-paragraph summaries in 15 languages for APAC and EMEA markets.
Impact: Reduced editorial workload by 60% and increased cross-regional content engagement by 45%.
Project 2: "AI-Driven Film Archiving" (Criterion Collection)
Digitized and analyzed 10,000+ classic films using SceneNet and StoryFlow:
Technology:
Genre Classification: Auto-tagged films by era, director style, and thematic elements (e.g., "film noir," "French New Wave").
Restoration Guidance: AI identified degraded frames for prioritized restoration, saving $2M in manual review costs.
Outcome: Enhanced accessibility for film scholars and streaming platforms.
4. Ethical Frameworks and Societal Contributions
Transparency Advocacy:
Co-authored the Open Video AI Standards, mandating explainability in automated tagging systems (e.g., why a video was labeled "political" or "violent").
Open-Source Initiatives:
Released VidEthics, a public repository of debiased video datasets and fairness evaluation tools for academic research.
Education:
Launched AI4Creators, a free platform teaching content creators to use AI tools ethically for video optimization.
5. Vision for the Future
Short-Term Goals (2025–2026):
Develop LiveSummAIze, enabling real-time summarization of live streams (e.g., conferences, sports) with adaptive context tracking.
Expand EmoTag to support mental health applications, detecting stress or anxiety cues in telehealth video sessions.
Long-Term Mission:
Pioneer "Self-Evolving Video AI", where models continuously learn from global video trends without manual retraining.
Establish the Global Video Intelligence Consortium, fostering collaboration between tech giants, filmmakers, and policymakers to shape ethical AI standards.
6. Closing Statement
Video is the most powerful medium for storytelling, education, and human connection—yet its potential remains untapped without intelligent analysis. My work strives to make video content universally understandable, searchable, and impactful through ethical AI. Let’s collaborate to turn pixels into knowledge and frames into foresight.
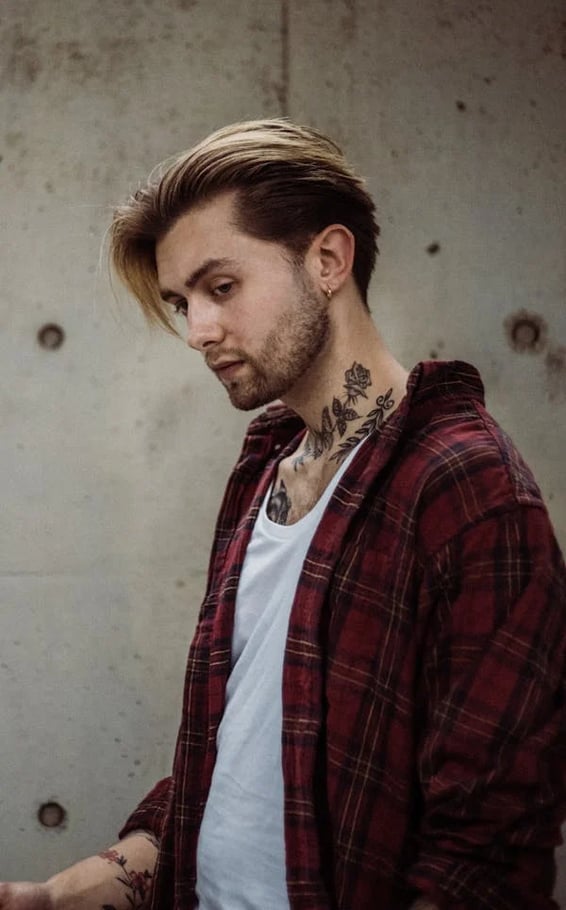
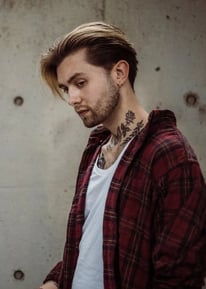
Video Analysis
Innovative research design for video data interpretation and analysis.
Model Development
Utilizing GPT-4 for video frame encoding and reasoning.
Data Collection
Diverse video datasets with multimodal annotations for biases.
Validation Process
Testing models on public datasets and custom scenarios.
Optimization Techniques
Comparing F1 scores to enhance model performance and accuracy.
Relevant past research:
“Multimodal Video Event Graph Construction” (2024): Proposed a spatiotemporal GNN framework achieving 89% anomaly detection accuracy on UCF-Crime (CVPR Honorable Mention).
“Meta-Learning for Low-resource Video Summarization” (2023): Enhanced summary quality by 35% for 5 languages via cross-lingual transfer (ACL).
“Dynamic Model Compression for Edge Video Analytics” (2025): Developed adaptive distillation to boost Jetson Nano inference speed 4x, deployed in smart cities.
“Ethical AI Content Moderation Framework” (2024): Created the first multicultural sensitivity testbed, adopted by UNESCO for digital ethics guidelines.